20 December 2007 to 4 October 2007
¶ A Report from Japan · 20 December 2007
Not a lot of activity on my discussion board recently, or really ever, but at my urging Ryan Allaer just posted a really good report from his music observation-post in Japan, so if you've stopped checking vF, now would be a good time to briefly start again!
¶ Music Never Loses · 19 November 2007
The New England Revolution lost the 2007 MLS Cup final yesterday, 2-1. They made it to the final and lost last year, too. And the year before that. And in 2002, as well, when I was there in person to see it. Four times, and each time it makes me feel terrible. I want to be able to change the world and make the losses not have occurred, and barring that I want to not have to interact with any other human being for about a week. The premise, I think, is that the potential joy of winning, or actually the present-value of the future joy of one day possibly winning, outweighs the pain of losing, no matter how many times multiplied. Maybe this math works, but don't ask me to check it from experience until next season is underway.
The MLS Cup is not yet a media circus on the scale of the championships of more-established domestic sports, but I still don't expect to care about the halftime show. This year, though, it was a brief live performance by Jimmy Eat World. Only little bits were shown on the TV broadcast, but that was enough to make me happy, even if the Revolution hadn't been leading at halftime.
A day later, the Revs loss is no longer as numbing or present, but it is still a source of pain. But Chase This Light, the new Jimmy Eat World album, is wonderful. It was wonderful last week, it's wonderful this week. For some reason I can't rationally identify, it sings to me of Winter in a glittery, embracing, light-and-hope-drenched way. It makes me love frailty more, and love more.
By the next time the Revolution try to win a championship, my daughter will be old enough to understand, if not the event, then what it does to me. She will learn to care about things that can hurt her, or she won't. This will be her decision, not mine.
But she can already dance. She can already see what these songs do to me. Music does not compete. Chase This Light does not defeat Clarity or Futures, never mind Odinist or American Doll Posse or Send Away the Tigers. Loss is not required. It is possible to love everything more than everything else. I give her a world in which this is true, and this time I don't have to wish I could change anything, because this truth is already true here.
The MLS Cup is not yet a media circus on the scale of the championships of more-established domestic sports, but I still don't expect to care about the halftime show. This year, though, it was a brief live performance by Jimmy Eat World. Only little bits were shown on the TV broadcast, but that was enough to make me happy, even if the Revolution hadn't been leading at halftime.
A day later, the Revs loss is no longer as numbing or present, but it is still a source of pain. But Chase This Light, the new Jimmy Eat World album, is wonderful. It was wonderful last week, it's wonderful this week. For some reason I can't rationally identify, it sings to me of Winter in a glittery, embracing, light-and-hope-drenched way. It makes me love frailty more, and love more.
By the next time the Revolution try to win a championship, my daughter will be old enough to understand, if not the event, then what it does to me. She will learn to care about things that can hurt her, or she won't. This will be her decision, not mine.
But she can already dance. She can already see what these songs do to me. Music does not compete. Chase This Light does not defeat Clarity or Futures, never mind Odinist or American Doll Posse or Send Away the Tigers. Loss is not required. It is possible to love everything more than everything else. I give her a world in which this is true, and this time I don't have to wish I could change anything, because this truth is already true here.
¶ great communications skills, but more importantly... · 8 November 2007
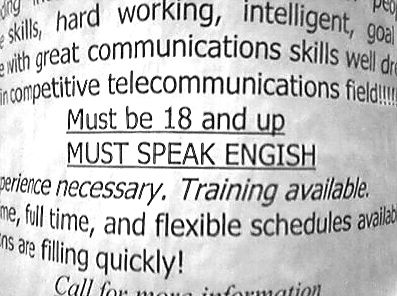
¶ The Evil Hiding in These Dead Trees · 25 October 2007 essay/tech
There are no dead-ends in data. Everything connects to something, and if anybody tells you otherwise, you should suspect them of hoping you won't figure out the connection they've omitted.
But we have suffered, for most of humanity's life with data, without good tools for really recognizing the connectivity of all things. We have cut down trees of wood and used them to make trees of data. Trees are full of dead-ends, of narrower and narrower branches. Books are mostly trees. Documents are usually trees. Spreadsheets tend to be trees. Speeches are trees. Trees say "We built 5 solar-power plants this year", and you either trust them, or else you break off the end of the branch and go looking for some other tree this branch could have come from. Trees are ways of telling stories that yearn constantly to end, of telling stories you can circumscribe, and saw through, and burn.
And stories you can cut down and burn are Evil's favorite medium. Selective partial information is ignorance's fastest friend. They built 5 solar plants, they say. Is that right? And how big were they? And where? And why did they say "we built", past-tense, not "we are now running"? And how many of last year's 5 did they close this year? And how many shoddy coal plants did they bolt together elsewhere, while the PR people were shining the sun in our eyes? There are statements, and then there are facts, and then there is Truth; and Truth is always tied up in the connections.
Not that we haven't ever tried to fix this, of course. Indices help. Footnotes help. Dictionaries and encyclopedias and catalogs help. Librarians help. Archivists and critics and contrarians and journalists help. Anything helps that lets assertions carry their context, and makes conclusions act always also as beginnings. Human diligence can weave the branches back together a little, knit the trees back into a semblance of the original web of knowledge. But it takes so much effort just to keep from losing what we already knew, effort stolen from time to learn new things, from making connections we didn't already throw away.
The Web helps, too, by giving us in some big ways the best tools for connection that we've ever had. Now your assertions can be packaged with their context, at least loosely and sometimes, if you make the effort. Now unsupported conclusions can be, if nothing else, terms for the next Google or Wikipedia search. This is more than we had before. It is a little harder for Evil to hide now, harder to lie and get away with it, harder to control the angle from which you don't see the half-truth's frayed ends.
But these are all still ultimately tenuous triumphs of constant human vigilance. The machines don't care what we say. The machines do not fact-check or cross-reference, of their own volition, and only barely help us when we try to do the work ourselves. The Web ought to be a web, a graph, but mostly it's just more trees. Mostly, any direction you crawl, you keep ending up on the narrowest branches, listening for the crack. All the paths of Truth may exist somewhere, but that doesn't mean you can follow them from any particular here to any specific there.
And even if linking were thoroughly ubiquitous, and most of the Web weren't SQL dumps occasionally fogged in by tag clouds, this would still be far from enough. The links alone are nowhere near enough, and believing they are is selling out this revolution before it has deposed anything, before it has done much more than make some posters. It is not enough for individual assertions to carry their context. It is not enough for our vocabulary of connection to be reduced to "see also", even if that temporarily seems like an expansion. It is not enough to link the self-aggrandizing press-release about solar plants to the company's web site, and hope you can find their SEC filings under Investor Relations somewhere. It is not enough to link the press-release to the filings, or for your blog-post about their operations in China to make Digg for six hours, or to take down one company or expose one lie. We've built a system that fountains half-truths at an unprecedented speed, and it is nowhere near enough to complete the half-truths one at a time.
The real revolution in information consists of two fundamental changes, neither of which have really begun yet in anything like the pervasive way they must:
1. The standard tools and methods for representing and presenting information must understand that everything connects, that "information" is mostly, or maybe exactly, those connections. As easy as it once became to print a document, and easier than it has become to put up web-pages and query-forms and database results-lists, it must become to describe and create and share and augment sets of data in which every connection, from every point in every direction, is inherently present and plainly evident. Not better tools for making links, tools that understand that the links are already inextricably everywhere.
2. The standard tools for exploring and consuming and analyzing connected information must move far beyond dealing with the connections one at a time. It is not enough to look up the company that built those plants. It is not enough to look up each of their yearly financial reports, one by one, for however many years you have patience to click. It's time to let the machines actually help us. They've been sitting around mostly wasting their time ever since toasters started flying, and we can't afford that any more. We need to be able to ask "What are the breakdowns of spending by plant-type for all companies that have built solar plants?", and have the machines go do all the clicking and collating and collecting. Otherwise our fancy digital web-pages might as well be illuminated manuscripts in bibliographers' crypts for all the good they do us. Linked pages must give way to linked data even more sweepingly and transformationally than shelved documents have given way to linked pages.
And because we can't afford to wait until machines learn to understand human languages, we will have to begin by speaking to them like machines, like we aren't just hoping they'll magically become us. We will have to shift some of our attention, at least some of us some of the time, from writing sentences to binding fields to actually modeling data, and to modeling the tools for modeling data. From Google to Wikipedia to Freebase, from search terms to query languages to exploration languages, from multimedia to interactive to semantic, from commerce to community to evolving insight. We have not freed ourselves from the tyranny of expertise, we've freed expertise from the obscurity of stacks. Escaping from trees is not escaping from structure, it is freeing structure. It is bringing alive what has been petrified.
There are no dead-ends in knowledge. Everything we know connects, by definition. We connect it by knowing. We connect. This is what we do, and thus what we must do better, and what we must train and allow our tools to help us do, and the only way Truth ever defeats Evil. Connecting matters. Truths, tools, links, schemata, graph alignment, ontology, semantics, inference: these things matter. The internet matters. This is why the internet matters.
But we have suffered, for most of humanity's life with data, without good tools for really recognizing the connectivity of all things. We have cut down trees of wood and used them to make trees of data. Trees are full of dead-ends, of narrower and narrower branches. Books are mostly trees. Documents are usually trees. Spreadsheets tend to be trees. Speeches are trees. Trees say "We built 5 solar-power plants this year", and you either trust them, or else you break off the end of the branch and go looking for some other tree this branch could have come from. Trees are ways of telling stories that yearn constantly to end, of telling stories you can circumscribe, and saw through, and burn.
And stories you can cut down and burn are Evil's favorite medium. Selective partial information is ignorance's fastest friend. They built 5 solar plants, they say. Is that right? And how big were they? And where? And why did they say "we built", past-tense, not "we are now running"? And how many of last year's 5 did they close this year? And how many shoddy coal plants did they bolt together elsewhere, while the PR people were shining the sun in our eyes? There are statements, and then there are facts, and then there is Truth; and Truth is always tied up in the connections.
Not that we haven't ever tried to fix this, of course. Indices help. Footnotes help. Dictionaries and encyclopedias and catalogs help. Librarians help. Archivists and critics and contrarians and journalists help. Anything helps that lets assertions carry their context, and makes conclusions act always also as beginnings. Human diligence can weave the branches back together a little, knit the trees back into a semblance of the original web of knowledge. But it takes so much effort just to keep from losing what we already knew, effort stolen from time to learn new things, from making connections we didn't already throw away.
The Web helps, too, by giving us in some big ways the best tools for connection that we've ever had. Now your assertions can be packaged with their context, at least loosely and sometimes, if you make the effort. Now unsupported conclusions can be, if nothing else, terms for the next Google or Wikipedia search. This is more than we had before. It is a little harder for Evil to hide now, harder to lie and get away with it, harder to control the angle from which you don't see the half-truth's frayed ends.
But these are all still ultimately tenuous triumphs of constant human vigilance. The machines don't care what we say. The machines do not fact-check or cross-reference, of their own volition, and only barely help us when we try to do the work ourselves. The Web ought to be a web, a graph, but mostly it's just more trees. Mostly, any direction you crawl, you keep ending up on the narrowest branches, listening for the crack. All the paths of Truth may exist somewhere, but that doesn't mean you can follow them from any particular here to any specific there.
And even if linking were thoroughly ubiquitous, and most of the Web weren't SQL dumps occasionally fogged in by tag clouds, this would still be far from enough. The links alone are nowhere near enough, and believing they are is selling out this revolution before it has deposed anything, before it has done much more than make some posters. It is not enough for individual assertions to carry their context. It is not enough for our vocabulary of connection to be reduced to "see also", even if that temporarily seems like an expansion. It is not enough to link the self-aggrandizing press-release about solar plants to the company's web site, and hope you can find their SEC filings under Investor Relations somewhere. It is not enough to link the press-release to the filings, or for your blog-post about their operations in China to make Digg for six hours, or to take down one company or expose one lie. We've built a system that fountains half-truths at an unprecedented speed, and it is nowhere near enough to complete the half-truths one at a time.
The real revolution in information consists of two fundamental changes, neither of which have really begun yet in anything like the pervasive way they must:
1. The standard tools and methods for representing and presenting information must understand that everything connects, that "information" is mostly, or maybe exactly, those connections. As easy as it once became to print a document, and easier than it has become to put up web-pages and query-forms and database results-lists, it must become to describe and create and share and augment sets of data in which every connection, from every point in every direction, is inherently present and plainly evident. Not better tools for making links, tools that understand that the links are already inextricably everywhere.
2. The standard tools for exploring and consuming and analyzing connected information must move far beyond dealing with the connections one at a time. It is not enough to look up the company that built those plants. It is not enough to look up each of their yearly financial reports, one by one, for however many years you have patience to click. It's time to let the machines actually help us. They've been sitting around mostly wasting their time ever since toasters started flying, and we can't afford that any more. We need to be able to ask "What are the breakdowns of spending by plant-type for all companies that have built solar plants?", and have the machines go do all the clicking and collating and collecting. Otherwise our fancy digital web-pages might as well be illuminated manuscripts in bibliographers' crypts for all the good they do us. Linked pages must give way to linked data even more sweepingly and transformationally than shelved documents have given way to linked pages.
And because we can't afford to wait until machines learn to understand human languages, we will have to begin by speaking to them like machines, like we aren't just hoping they'll magically become us. We will have to shift some of our attention, at least some of us some of the time, from writing sentences to binding fields to actually modeling data, and to modeling the tools for modeling data. From Google to Wikipedia to Freebase, from search terms to query languages to exploration languages, from multimedia to interactive to semantic, from commerce to community to evolving insight. We have not freed ourselves from the tyranny of expertise, we've freed expertise from the obscurity of stacks. Escaping from trees is not escaping from structure, it is freeing structure. It is bringing alive what has been petrified.
There are no dead-ends in knowledge. Everything we know connects, by definition. We connect it by knowing. We connect. This is what we do, and thus what we must do better, and what we must train and allow our tools to help us do, and the only way Truth ever defeats Evil. Connecting matters. Truths, tools, links, schemata, graph alignment, ontology, semantics, inference: these things matter. The internet matters. This is why the internet matters.
It is possible for even the most preternaturally precocious child to actually miss a diaper from one tenth of an inch away.
Here is a gift, of unspecified value, to the field of set-comparison math: The Empath Coefficient, an alternate measure of the alignment between two sets. Conceptually this is intended as a rough proxy for measuring the degree to which the unseen or impractical-to-measure motivation behind the membership of set A also informs the membership of set B, but the math is what it is, so the next time you find yourself comparing the Cosine, Dice and Tanimoto coefficients, looking for something faster than TF-IDF to make some sense of your world, here's another thing to try. This is the one I used in empath, my recent similarity-analysis of heavy-metal bands, if you want to see lots of examples of it in action.
At its base, the Empath Coefficient is an asymmetric measure, based on the idea that in a data distribution with some elements that appear in many sets and some that appear in only a few, it is not very interesting to discover that everything is "similar" to the most-popular things. E.g., "People who bought Some Dermatological Diseases of the Domesticated Turtle also bought Harry Potter and the...". In the Empath calculation, then, the size of the Harry Potter set (the one you're comparing) affects the similarity more than the size of the Turtle set (the one you're trying to learn about). I have arrived at a 1:3 weighting through experimenting with a small number of data-sets, and do not pretend to offer any abstract mathmatical justification for this ratio, so if you want to parameterize the second-set weight and call that the Npath Coefficient, go ahead.
Where the Dice Coefficient, then, divides the size of the overlap by the average size of the two sets (call A the size of the first set, B the size of the second set, and V the size of the overlap):
the core of the Empath Coefficient adjusts this to:
By itself, though, that calculation will still be uninformatively dominated by small overlaps between small sets, so I further discount the similarities based on the overlap size. Like this:
So if the overlap size (V) is only 1, the core score is multiplied by 1/2 [1-1/(1+1)], if it's 2 the core score is multiplied by 2/3 [1-1/(2+1)], etc. And then, for good measure, I parameterize the whole thing to allow the assertion of a minimum overlap size, M, which goes into the adjustment numerator like this:
This way the sample-size penalties are automatically calibrated to the threshold, and below the threshold the scores actually go negative. You can obviously overlay a threshold on the other coefficients in pre- or post-processing, but I think it's much cooler to have the math just take care of it.
I also sometimes use another simpler asymmetric calculation, the Subset Coefficient, which produces very similar rankings to Empath's for any given A against various Bs (especially if the sets are all large):
The concept here is that we take A as stipulated, and then compare B to A's subset of B, again deducting points for small sample-sizes. The biggest disadvantage of Subset is that scores for As of different sizes are not calibrated against each other, so comparing A1/B1 similarity to A2/B2 similarity won't necessarily give you useful results. But sometimes you don't care about that.
This is the one I used for calculating artist clusters from 2006 music-poll data, where cross-calibration was inane to worry about because the data was so limited to begin with.
Here, then, are the forumlae for all five of these coefficients:
Cosine: V/sqrt(AB)
Dice: 2V/(A+B)
Tanimoto: V/(A+B-V)
Subset: (V-1)/B
Empath: 4V(1-M/(V+1))/(A+3B)
And here are some example scores and ranks:
A few things to note:
- In 1 & 2, notice that Dice, Tanimoto and Cosine all produce 1.0 scores for congruent sets, no matter what their size. Subset and Empath only approach 1, and give higher scores to larger sets. The idea is that the larger the two sets are, the more unlikely it is that they coincide by chance.
- 5 & 6, 7 & 8 and 11 & 12 are reversed pairs, so you can see how the two asymmetric calculations handle them.
- Empath produces the finest granularity of scores, by far, including no ties even within this limited set of examples. Whether this is good or bad for any particular data-set of yours is up to you to decide.
- Since all of these work with only the set and overlap sizes, none of them take into account the significance of two sets overlapping at some specific element. If you want to probability-weight, to say that sharing a seldom-shared element is worth more than sharing an often-shared element, then look up term frequency -- inverse document frequency, and plan to spend more calculation cycles. Sometimes you need this. (I used tf-idf for comparing music-poll voters, where the set of set-sizes was so small that without taking into account the popularity/obscurity of the albums on which voters overlapped, you couldn't get any interesting numbers at all.)
There may or may not be some clear mathematical way to assess the fitness of each of these various measurements for a given data-set, based on its connectedness and distribution, but at any rate I am not going to provide one. If you actually have data with overlapping sets whose similarity you're trying to measure, I suggest trying all five, and examining their implications for some corner of your data you personally understand, where you yourself can meta-evaluate the scores and rankings that the math produces. I do not contend that my equations produce more-objective truths than the other ones; only that the stories they tell me about things I know are plausible, and the stories they have told me about things I didn't know have usually proven to be interesting.
At its base, the Empath Coefficient is an asymmetric measure, based on the idea that in a data distribution with some elements that appear in many sets and some that appear in only a few, it is not very interesting to discover that everything is "similar" to the most-popular things. E.g., "People who bought Some Dermatological Diseases of the Domesticated Turtle also bought Harry Potter and the...". In the Empath calculation, then, the size of the Harry Potter set (the one you're comparing) affects the similarity more than the size of the Turtle set (the one you're trying to learn about). I have arrived at a 1:3 weighting through experimenting with a small number of data-sets, and do not pretend to offer any abstract mathmatical justification for this ratio, so if you want to parameterize the second-set weight and call that the Npath Coefficient, go ahead.
Where the Dice Coefficient, then, divides the size of the overlap by the average size of the two sets (call A the size of the first set, B the size of the second set, and V the size of the overlap):
V/((A+B)/2)
or
2V/(A+B)
the core of the Empath Coefficient adjusts this to:
V/((A+3B)/4)
or
4V/(A+3B)
By itself, though, that calculation will still be uninformatively dominated by small overlaps between small sets, so I further discount the similarities based on the overlap size. Like this:
(1-1/(V+1)) * V/((A+3B)/4)
or
4V(1-1/(V+1))/(A+3B)
So if the overlap size (V) is only 1, the core score is multiplied by 1/2 [1-1/(1+1)], if it's 2 the core score is multiplied by 2/3 [1-1/(2+1)], etc. And then, for good measure, I parameterize the whole thing to allow the assertion of a minimum overlap size, M, which goes into the adjustment numerator like this:
4V(1-M/(V+1))/(A+3B)
This way the sample-size penalties are automatically calibrated to the threshold, and below the threshold the scores actually go negative. You can obviously overlay a threshold on the other coefficients in pre- or post-processing, but I think it's much cooler to have the math just take care of it.
I also sometimes use another simpler asymmetric calculation, the Subset Coefficient, which produces very similar rankings to Empath's for any given A against various Bs (especially if the sets are all large):
(V-1)/B
The concept here is that we take A as stipulated, and then compare B to A's subset of B, again deducting points for small sample-sizes. The biggest disadvantage of Subset is that scores for As of different sizes are not calibrated against each other, so comparing A1/B1 similarity to A2/B2 similarity won't necessarily give you useful results. But sometimes you don't care about that.
This is the one I used for calculating artist clusters from 2006 music-poll data, where cross-calibration was inane to worry about because the data was so limited to begin with.
Here, then, are the forumlae for all five of these coefficients:
Cosine: V/sqrt(AB)
Dice: 2V/(A+B)
Tanimoto: V/(A+B-V)
Subset: (V-1)/B
Empath: 4V(1-M/(V+1))/(A+3B)
And here are some example scores and ranks:
# | A | B | V | Dice | Rank | Tanimoto | Rank | Cosine | Rank | Subset | Rank | Empath | Rank |
1 | 100 | 100 | 100 | 1.000 | 1 | 1.000 | 1 | 1.000 | 1 | 0.990 | 1 | 0.990 | 1 |
2 | 10 | 10 | 10 | 1.000 | 1 | 1.000 | 1 | 1.000 | 1 | 0.900 | 2 | 0.909 | 2 |
3 | 10 | 10 | 5 | 0.500 | 3 | 0.333 | 3 | 0.500 | 5 | 0.400 | 4 | 0.417 | 4 |
4 | 10 | 10 | 2 | 0.200 | 12 | 0.111 | 12 | 0.200 | 12 | 0.100 | 11 | 0.133 | 12 |
5 | 10 | 5 | 3 | 0.400 | 6 | 0.250 | 6 | 0.424 | 6 | 0.400 | 5 | 0.360 | 5 |
6 | 5 | 10 | 3 | 0.400 | 6 | 0.250 | 6 | 0.424 | 6 | 0.200 | 8 | 0.257 | 8 |
7 | 10 | 5 | 2 | 0.267 | 10 | 0.154 | 10 | 0.283 | 10 | 0.200 | 7 | 0.213 | 10 |
8 | 5 | 10 | 2 | 0.267 | 10 | 0.154 | 10 | 0.283 | 10 | 0.100 | 11 | 0.152 | 11 |
9 | 6 | 6 | 2 | 0.333 | 9 | 0.200 | 9 | 0.333 | 9 | 0.167 | 9 | 0.222 | 9 |
10 | 6 | 4 | 2 | 0.400 | 6 | 0.250 | 6 | 0.408 | 8 | 0.250 | 6 | 0.296 | 6 |
11 | 6 | 2 | 2 | 0.500 | 3 | 0.333 | 3 | 0.577 | 3 | 0.500 | 3 | 0.444 | 3 |
12 | 2 | 6 | 2 | 0.500 | 3 | 0.333 | 3 | 0.577 | 3 | 0.167 | 9 | 0.267 | 7 |
A few things to note:
- In 1 & 2, notice that Dice, Tanimoto and Cosine all produce 1.0 scores for congruent sets, no matter what their size. Subset and Empath only approach 1, and give higher scores to larger sets. The idea is that the larger the two sets are, the more unlikely it is that they coincide by chance.
- 5 & 6, 7 & 8 and 11 & 12 are reversed pairs, so you can see how the two asymmetric calculations handle them.
- Empath produces the finest granularity of scores, by far, including no ties even within this limited set of examples. Whether this is good or bad for any particular data-set of yours is up to you to decide.
- Since all of these work with only the set and overlap sizes, none of them take into account the significance of two sets overlapping at some specific element. If you want to probability-weight, to say that sharing a seldom-shared element is worth more than sharing an often-shared element, then look up term frequency -- inverse document frequency, and plan to spend more calculation cycles. Sometimes you need this. (I used tf-idf for comparing music-poll voters, where the set of set-sizes was so small that without taking into account the popularity/obscurity of the albums on which voters overlapped, you couldn't get any interesting numbers at all.)
There may or may not be some clear mathematical way to assess the fitness of each of these various measurements for a given data-set, based on its connectedness and distribution, but at any rate I am not going to provide one. If you actually have data with overlapping sets whose similarity you're trying to measure, I suggest trying all five, and examining their implications for some corner of your data you personally understand, where you yourself can meta-evaluate the scores and rankings that the math produces. I do not contend that my equations produce more-objective truths than the other ones; only that the stories they tell me about things I know are plausible, and the stories they have told me about things I didn't know have usually proven to be interesting.
¶ How You Win · 4 October 2007
My favorite moment in the New England Revolution's 3-2 defeat of FC Dallas for the 2007 U.S. Open Cup is not unheralded rookie Wells Thompson beating semi-heralded international Adrian Serioux to the ball and slipping it past almost-semi-heralded international Dario Sala for what ends up being the game-winning goal. It is not once-unheralded-rookie Pat Noonan's no-look back-flick into Thompson's trailing run. It is not once-unheralded-rookie Taylor Twellman's pinpoint cross to Noonan's feet. It is before that, as Twellman and Noonan work forward, and Noonan's pass back towards Twellman goes a little wide. As he veers to chase it down, Twellman gives one of his little chugging acceleration moves, a tiny but unmistakable physical manifestation of his personality. I've watched him do this for years. I can recognize him out of the corner of my eye on a tiny TV across a crowded room, just from how he runs. Just from how he steps as he runs.
This is the Revolution's first trophy, after losing three MLS Cups and one prior Open Cup, all in overtime or worse. They are my team because I live here, that's how sports fandom mainly works. But I care about them, not just support them, because Steve Nicol runs the team with deliberate atavistic moral clarity. The Revs develop players. Of the 12 players who appeared in this victory, 8 were Revolution draft picks, 1 was a Revolution discovery player, 2 of the remaining 3 were acquired in trades before Nicol took over, and the last one (Matt Reis) was acquired in an off-season trade before Nicol's first season even started. Of the 5 other field-players on the bench last night, even, 3 are Nicol draft-picks and other 2 are Revs discoveries. As is Shalrie Joseph, suspended for a red-card picked up in the semi-final during an altercation with, ironically enough, yet another Revolution draft-pick now playing in the USL. Even the Revs' misfortunes are products of their own dedication.
Arguably other teams, using more opportunistic methods, have acquired better players. Several of them have acquired more trophies. But none of them, I think, are more coherently themselves. None of them can hold up a trophy and know that they earned it, as a self-contained organization, this completely.
Sometimes, as a sports fan, you get to be happy. Much more rarely, you get to be proud.
This is the Revolution's first trophy, after losing three MLS Cups and one prior Open Cup, all in overtime or worse. They are my team because I live here, that's how sports fandom mainly works. But I care about them, not just support them, because Steve Nicol runs the team with deliberate atavistic moral clarity. The Revs develop players. Of the 12 players who appeared in this victory, 8 were Revolution draft picks, 1 was a Revolution discovery player, 2 of the remaining 3 were acquired in trades before Nicol took over, and the last one (Matt Reis) was acquired in an off-season trade before Nicol's first season even started. Of the 5 other field-players on the bench last night, even, 3 are Nicol draft-picks and other 2 are Revs discoveries. As is Shalrie Joseph, suspended for a red-card picked up in the semi-final during an altercation with, ironically enough, yet another Revolution draft-pick now playing in the USL. Even the Revs' misfortunes are products of their own dedication.
Arguably other teams, using more opportunistic methods, have acquired better players. Several of them have acquired more trophies. But none of them, I think, are more coherently themselves. None of them can hold up a trophy and know that they earned it, as a self-contained organization, this completely.
Sometimes, as a sports fan, you get to be happy. Much more rarely, you get to be proud.